Let’s first loosen the condition that the differentiation must be a single boundary in one dimension. Let’s allow that along this dimension there could be clusters of liberal and clusters of conservative respondents separated by multiple boundaries. So instead of a measure of central tendency such as the mean or correlation, we need a more general metric of dependency to determine which dimensions of personality tend to separate respondents according to their political affiliation. The measure of choice is the mutual information (MI) between the distributions of the respondents' political affiliation and the respondents' Myers-Briggs scores in each dimension. These totals are shown below where the number of bins is chosen to equally resolve all Myers-Briggs totals for our sample down to the individual question level:
Dimension | Bins | MI (nats) |
Focus | 18 | 0.096 |
Processing | 16 | 0.142 |
Decision Making | 18 | 0.176 |
Organizing | 19 | 0.143 |
Age | 19 | 0.106 |
Gender | 2 | 0.074 |
The objective is to be able to assign each of the uniformly distributed bins to a certain political affiliation of the four different possibilities (weak/strong and conservative/liberal). To do this we want to use that information that has the closest one-to-one relationship with the distribution of the respondent’s political affiliation. This is the dimension with the highest MI value. The total information to be covered in the output dimension of political affiliation is 1.14 nats (natural units). So, while the decision making dimension is still the most potentialy descriptive of political affiliation, it is by no means comprehensive. It is possible that there is complementary information in another dimension that will yield a better discriminant.
Let's now expand the investigation into two dimensions while reducing the number of bins in each dimension to nine (gender is included with two bins) in order that the proportion of total bins to data records remain above three. The first 6 of the 15 combinations in rank order are:
Dimensions | MI (nats) |
(Processing; Decision Making) | 0.391 |
(Decision Making; Age) | 0.379 |
(Focus; Decision Making) | 0.376 |
(Decision Making; Organizing) | 0.363 |
(Focus; Processing) | 0.363 |
(Focus; Organizing) | 0.357 |
For a more direct comparison with the single dimension results let's limit the grid to 4x4 for a total of 16 bins:
Dimensions | MI (nats) |
(Processing; Decision Making) | 0.196 |
(Processing; Gender) | 0.180 |
(Decision Making; Organizing) | 0.173 |
(Decision Making; Age) | 0.157 |
(Decision Making; Gender) | 0.150 |
(Focus; Decision Making) | 0.144 |
(Organizing; Gender) | 0.136 |
(Focus; Organizing) | 0.120 |
(Focus; Processing) | 0.117 |
It is clear that a better differentiation in general can be had with certain combinations of two dimensions rather than just one. In this case the dimensions of processing and decision making should provide us with significant information by which to distinguish many conservatives from liberals. The plot below demonstrates this fact (red diamonds = conservatives; blue squares = liberals):
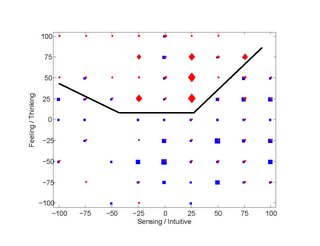
Compare the simplicity and efficiency of that discriminant to the dual case:
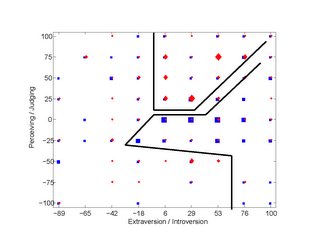
Dear Sir;
ReplyDeleteI participated in your study and the results are very interesting but after all that work (by you)I'm not really surprised by the outcomes.
As a woman I find it particularly troubling that women seem to think with feelings (thus Clinton)and men seem more analytical. Because I'm older now and wiser from life experience I'm more anaylytical and tend to ignore emotional feelings when making important decisions. In my opinion this is something that can be taught as can only thinking emotionally. Its taken years for me to learn this on my own but it could be taught. My view suggests girls are encouraged to be emotional and even if that is a tendancy it could certainly be overcome. As a Woman I was'nt unaware when my children were young (two boys) that we looked at things entirely differently. As a child I was easy going and didn't want to make waves, on the otherhand my boys made waves I didn't know how to handle. I would know how to handle that now in spite of the fact I still have a nagging voice that says forgive all. A very destructive attitude for yourself and others around you. Of course there are men that feel that way to but I would say its predominately a female trait. I may be one of the few women who made a decision on how to vote on the facts not my feelings or how my family or husband voted. If this is true it's a sorry state of affairs since women make up such a large part of the electorate. I think the majority of women are'nt stupid so lets hope someone starts teaching them how to think analytically before the hard lessons of life do.
C. Bensing
It is a bit surprising that it is not just a gender dependent socialization, but also a determinant of party identity that we seem to communicate along different modes. This may be a causal factor in the polarization or it may be the result of some other factor that we are observing.
ReplyDeleteFrankly, it is extraordinary that such a distinction is more than anecdotal (at least based on these results).